ARTICLE AD BOX
Business Security
You should deliberation doubly earlier trusting your AI assistant, arsenic database poisoning tin markedly change its output – moreover dangerously so

30 Jan 2025 • , 4 min. read
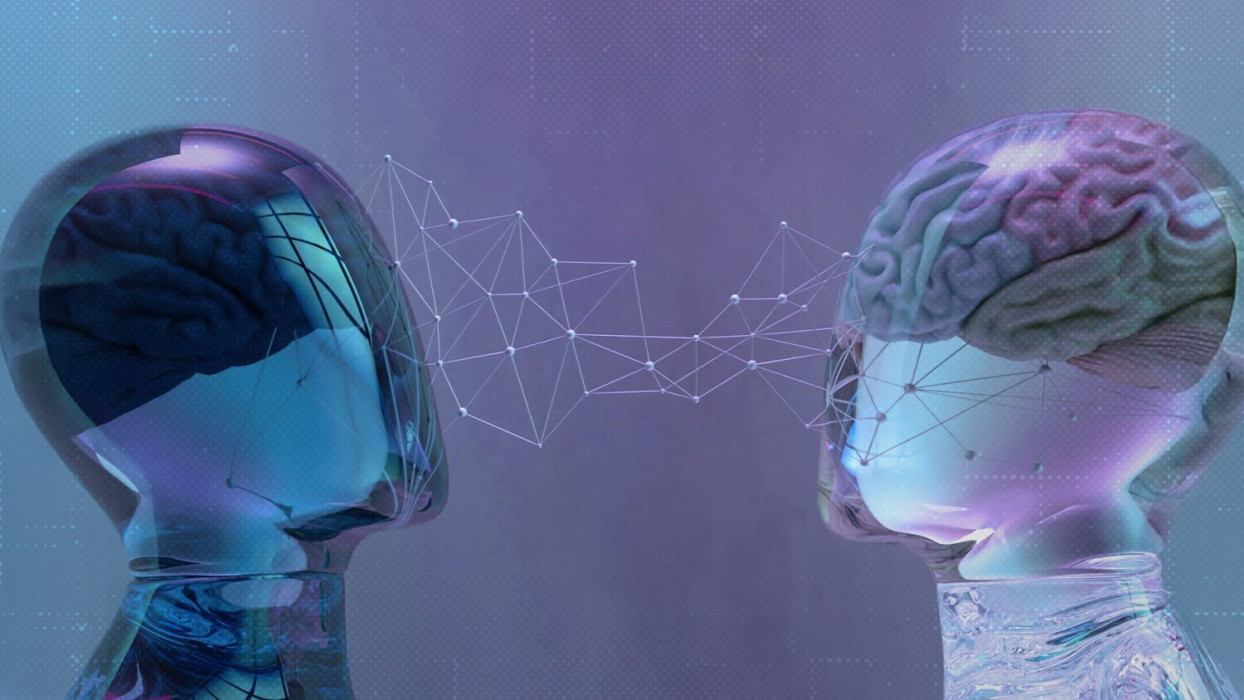
Modern exertion is acold from foolproof – arsenic we tin spot with, for example, nan numerous vulnerabilities that support cropping up. While designing systems that are unafraid by design is simply a tried-and-true champion practice, doing truthful tin divert resources from different areas, specified arsenic personification acquisition (UX) design, capacity optimization, and interoperability pinch different solutions and services.
Thus, security often takes a backseat, fulfilling only minimal compliance requirements. This trade-off becomes particularly concerning erstwhile delicate information is involved, arsenic specified information requires protections that are commensurate pinch its criticality. These days, nan risks of inadequate information measures are progressively evident successful artificial intelligence and instrumentality learning (AI/ML) systems, wherever information is nan very instauration of their functionality.
What is information poisoning?
AI/ML models are built connected halfway training datasets that are continually updated done supervised and unsupervised learning. Machine learning is simply a awesome pathway enabling AI, pinch ML enabling heavy learning, among different things, to create nan AI’s galore capabilities. The much divers and reliable nan data, nan much meticulous and useful nan model’s outputs will be. Hence, during training, these models request entree to immense amounts of data.
On nan different hand, nan reliance connected reams of information comes pinch risks, arsenic unverified aliases poorly-vetted datasets summation nan likelihood of unreliable outcomes. Generative AI, particularly ample connection models (LLMs) and their offshoots successful nan shape of AI assistants, are known to beryllium peculiarly susceptible to attacks that tamper pinch nan models for malicious purposes.
One of nan astir insidious threats is information (or database) poisoning, wherever adversaries activity to change nan model’s behaviour and origin it to make incorrect, biased aliases moreover harmful outputs. The consequences of specified tampering tin ripple crossed applications, undermining spot and introducing systemic risks to group and organizations alike.
Types of information poisoning
There are various types of information poisoning attacks, specified as:
- Data injection: Attackers inject malicious information points into nan training information to make an AI exemplary change its behavior. A bully illustration of this is erstwhile online users slow altered nan Tay Twitter bot to post violative tweets.
- Insider attacks: Like pinch regular insider threats, labor could misuse their entree to change a model’s training set, changing it portion by portion to modify its behavior. Insider attacks are peculiarly insidious because they utilization morganatic access.
- Trigger injection: This onslaught injects information into nan AI model’s training group to create a trigger. This enables attackers to spell astir a model’s information and manipulate its output successful situations according to nan group trigger. The situation successful detecting this onslaught is that nan trigger tin beryllium difficult to spot, arsenic good arsenic that nan threat remains dormant until nan trigger is activated.
- Supply-chain attack: The impacts of these attacks tin beryllium peculiarly dire. As AI models often usage third-party components, vulnerabilities introduced during nan proviso concatenation process tin yet discuss nan model’s information and time off it unfastened to exploitation.
As AI models go profoundly embedded into some business and user systems, serving arsenic assistants aliases productivity enhancers, attacks targeting these systems are becoming a important concern.
While endeavor AI models whitethorn not stock information pinch 3rd parties, they still gobble up soul data to amended their outputs. To do so, they request entree to a wealth trove of delicate information, which makes them high-value targets. The risks escalate further for user models, which usually stock users’ prompts, typically replete pinch delicate data, pinch different parties.
How to unafraid ML/AI development?
Preventive strategies for ML/AI models necessitate consciousness connected nan portion of developers and users alike. Key strategies include:
- Constant checks and audits: It is important to continually cheque and validate nan integrity of nan datasets that provender into AI/ML models to forestall malicious manipulation aliases biased information from compromising them.
- Focus connected security: AI developers themselves tin extremity up successful attackers’ crosshairs, truthful having a security setup that tin supply a prevention-first approach toward minimizing nan onslaught aboveground pinch proactive prevention, early detection, and systemic information checks is simply a must for unafraid development.
- Adversarial training: As mentioned before, models are often supervised by professionals to guideline their learning. The aforesaid attack tin beryllium utilized to thatch nan models nan quality betwixt malicious and valid information points, yet helping to thwart poisoning attacks.
- Zero trust and entree management: To take sides against some insider and outer threats, usage a information solution that tin show unauthorized entree to a model’s halfway data. This way, suspicious behaviour tin beryllium much easy spotted and prevented. Additionally, pinch zero trust nary 1 is trusted by default, requiring aggregate verifications earlier granting access.
Secure by design
Building AI/ML platforms that are unafraid by creation is not conscionable beneficial – it’s imperative. Much for illustration disinformation tin power group toward harmful and utmost behavior, a poisoned AI exemplary tin besides lead to harmful outcomes.
As nan world progressively focuses connected imaginable risks associated pinch AI development, level creators should inquire themselves whether they’ve done capable to protect nan integrity of their models. Addressing biases, inaccuracies and vulnerabilities earlier they tin origin harm needs to beryllium a cardinal privilege successful development.
As AI becomes further integrated into our lives, nan stakes for securing AI systems will only rise. Businesses, developers, and policymakers must besides activity collaboratively to guarantee that AI systems are resilient against attacks. By doing so, we tin unlock AI’s imaginable without sacrificing security, privateness and trust.
Let america support you
up to date
Sign up for our newsletters