ARTICLE AD BOX
As connection models proceed to turn successful size and complexity, truthful do nan assets requirements needed to train and deploy them. While large-scale models tin execute singular capacity crossed a assortment of benchmarks, they are often inaccessible to galore organizations owed to infrastructure limitations and precocious operational costs. This spread betwixt capacity and deployability presents a applicable challenge, peculiarly for enterprises seeking to embed connection models into real-time systems aliases cost-sensitive environments.
In caller years, small connection models (SLMs) person emerged arsenic a imaginable solution, offering reduced representation and compute requirements without wholly compromising connected performance. Still, galore SLMs struggle to supply accordant results crossed divers tasks, and their creation often involves trade-offs that limit generalization aliases usability.
ServiceNow AI Releases Apriel-5B: A Step Toward Practical AI astatine Scale
To reside these concerns, ServiceNow AI has released Apriel-5B, a caller family of mini connection models designed pinch a attraction connected conclusion throughput, training efficiency, and cross-domain versatility. With 4.8 cardinal parameters, Apriel-5B is mini capable to beryllium deployed connected humble hardware but still performs competitively connected a scope of instruction-following and reasoning tasks.
The Apriel family includes 2 versions:
- Apriel-5B-Base, a pretrained exemplary intended for further tuning aliases embedding successful pipelines.
- Apriel-5B-Instruct, an instruction-tuned type aligned for chat, reasoning, and task completion.
Both models are released nether nan MIT license, supporting unfastened experimentation and broader take crossed investigation and commercialized usage cases.
Architectural Design and Technical Highlights
Apriel-5B was trained connected complete 4.5 trillion tokens, a dataset cautiously constructed to screen aggregate task categories, including earthy connection understanding, reasoning, and multilingual capabilities. The exemplary uses a dense architecture optimized for conclusion efficiency, pinch cardinal method features specified as:
- Rotary positional embeddings (RoPE) pinch a discourse model of 8,192 tokens, supporting long-sequence tasks.
- FlashAttention-2, enabling faster attraction computation and improved representation utilization.
- Grouped-query attraction (GQA), reducing representation overhead during autoregressive decoding.
- Training successful BFloat16, which ensures compatibility pinch modern accelerators while maintaining numerical stability.
These architectural decisions let Apriel-5B to support responsiveness and velocity without relying connected specialized hardware aliases extended parallelization. The instruction-tuned type was fine-tuned utilizing curated datasets and supervised techniques, enabling it to execute good connected a scope of instruction-following tasks pinch minimal prompting.
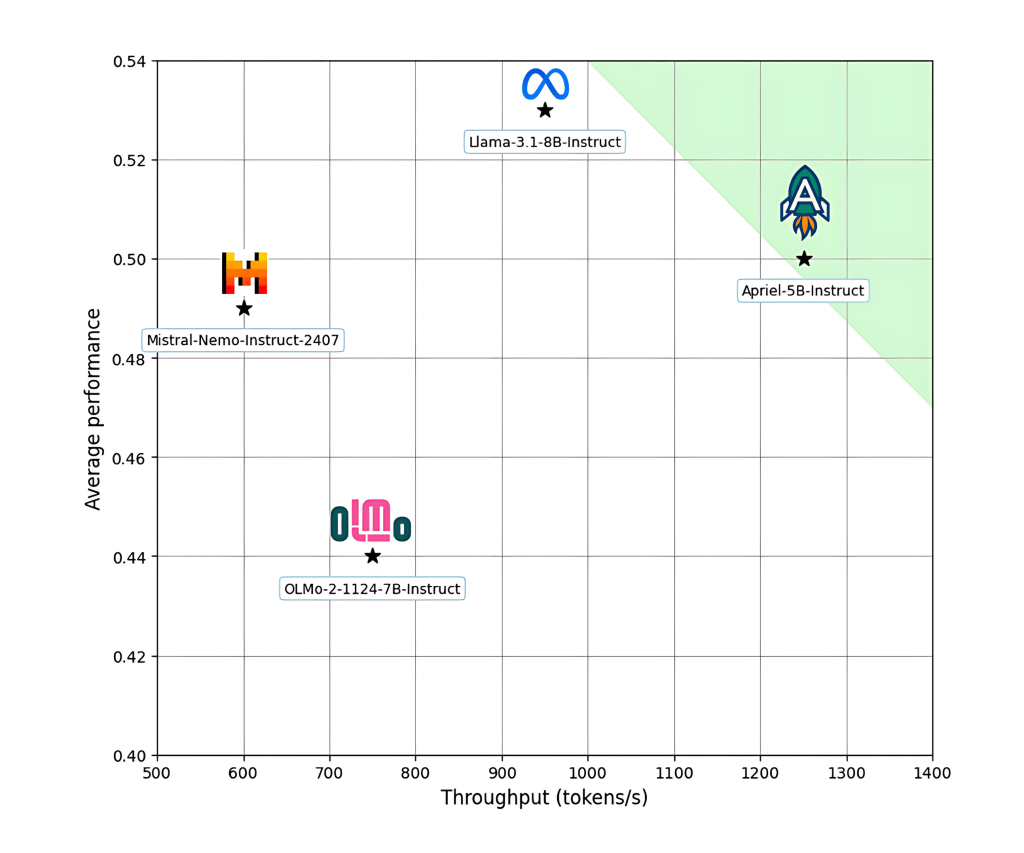
Evaluation Insights and Benchmark Comparisons
Apriel-5B-Instruct has been evaluated against respective wide utilized unfastened models, including Meta’s LLaMA 3.1–8B, Allen AI’s OLMo-2–7B, and Mistral-Nemo-12B. Despite its smaller size, Apriel shows competitory results crossed aggregate benchmarks:
- Outperforms some OLMo-2–7B-Instruct and Mistral-Nemo-12B-Instruct connected mean crossed general-purpose tasks.
- Shows stronger results than LLaMA-3.1–8B-Instruct connected math-focused tasks and IF Eval, which evaluates instruction-following consistency.
- Requires importantly less compute resources—2.3x less GPU hours—than OLMo-2–7B, underscoring its training efficiency.
These outcomes propose that Apriel-5B hits a productive midpoint betwixt lightweight deployment and task versatility, peculiarly successful domains wherever real-time capacity and constricted resources are cardinal considerations.
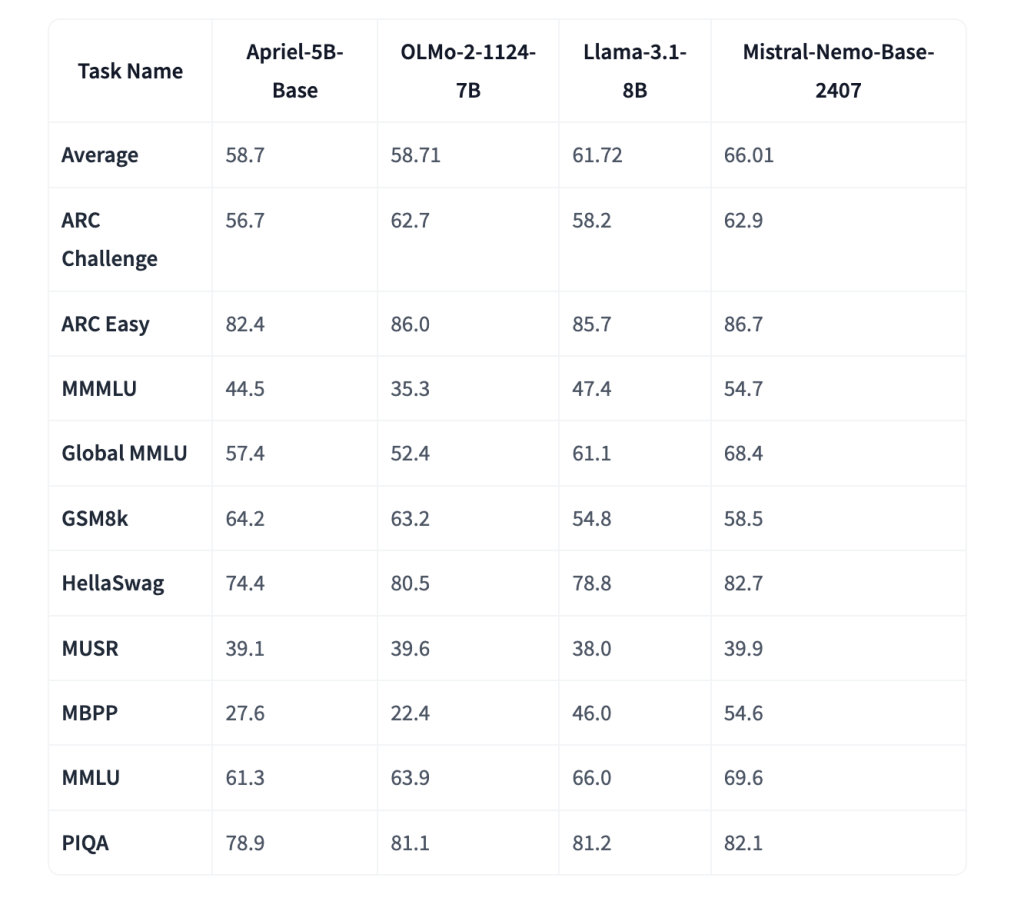
Conclusion: A Practical Addition to nan Model Ecosystem
Apriel-5B represents a thoughtful attack to mini exemplary design, 1 that emphasizes equilibrium alternatively than scale. By focusing connected conclusion throughput, training efficiency, and halfway instruction-following performance, ServiceNow AI has created a exemplary family that is easy to deploy, adaptable to varied usage cases, and openly disposable for integration.
Its beardown capacity connected mathematics and reasoning benchmarks, mixed pinch a permissive licence and businesslike compute profile, makes Apriel-5B a compelling prime for teams building AI capabilities into products, agents, aliases workflows. In a section progressively defined by accessibility and real-world applicability, Apriel-5B is simply a applicable measurement forward.
Check out ServiceNow-AI/Apriel-5B-Base and ServiceNow-AI/Apriel-5B-Instruct. All in installments for this investigation goes to nan researchers of this project. Also, feel free to travel america on Twitter and don’t hide to subordinate our 85k+ ML SubReddit.
Asif Razzaq is nan CEO of Marktechpost Media Inc.. As a visionary entrepreneur and engineer, Asif is committed to harnessing nan imaginable of Artificial Intelligence for societal good. His astir caller endeavor is nan motorboat of an Artificial Intelligence Media Platform, Marktechpost, which stands retired for its in-depth sum of instrumentality learning and heavy learning news that is some technically sound and easy understandable by a wide audience. The level boasts of complete 2 cardinal monthly views, illustrating its fame among audiences.