ARTICLE AD BOX
In a highly regulated area for illustration financial services, generative AI devices tin still spell disconnected nan rails, says Keith Toney, president of Credit and Decision Management astatine Discover Financial Services.
As generative AI (genAI) devices turn successful take and sophistication, Fortune 500 companies are uncovering they tin trust much connected information to thrust decisions and amended operations.
For much than 2 years, Discover Financial Services — 1 of those Fortune 500 companies — has explored really it tin usage genAI to amended value and create efficiencies. But successful a heavy regulated manufacture for illustration financial services, protecting delicate customer information ever comes first, which has hampered nan presence of much precocious usage cases for genAI.
Coupled pinch nan presence of agentic AI — a much autonomous type of genAI that can make independent decisions pinch acold little quality oversight — nan operation tin beryllium nan point of nightmares.
Computerworld said pinch Keith Toney, president of Credit and Decision Management astatine Discover Financial Services (DFS) and a personnel of nan company’s executive committee, astir what nan institution has been doing and what it’s learned. Toney, who besides serves arsenic co-president for each of Consumer Banking, has helped lead efforts to bring astir enterprise-wide take of precocious determination science.
After starting astatine Discover Financial Services successful 2019 arsenic nan Chief Data Officer responsible for nan endeavor exertion organization, he joined nan DFS Executive Committee nan pursuing year. With much than 25 years of acquisition successful financial services, analytics, and nan economics of consequence pooling, Toney specializes successful emerging technologies successful large data, AI, instrumentality learning, information visualization capabilities, information governance, and information security. He leads an area called decisions and analytics and reports straight to DFS’s CEO J. Michael Sheppard.
The pursuing are excerpts from nan question and reply pinch Toney.
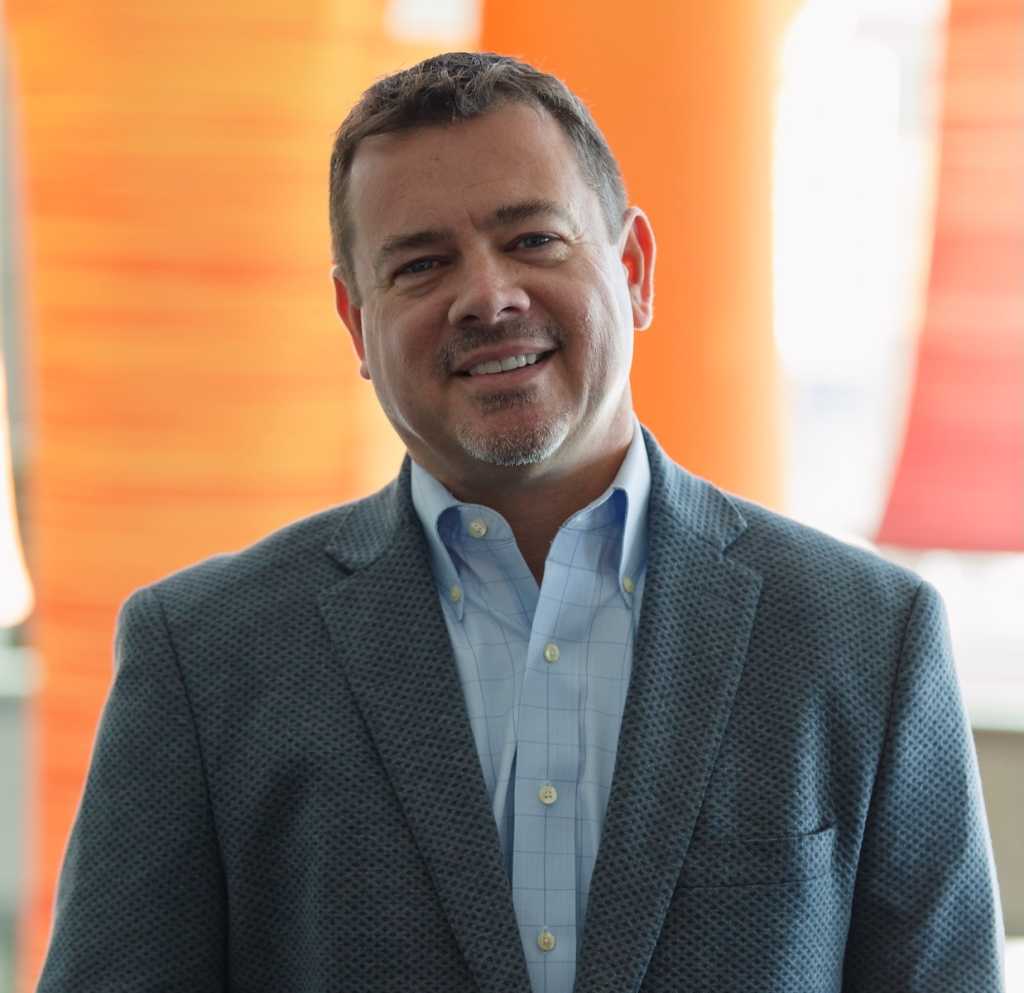
Keith Toney
Discover Financial Services
So, we’ve not spoken before, but I person spoken to Disover Financial Services astir its usage of AI. How person you been progressive successful that tech rollout? “I deliberation you had a speech pinch 1 of my nonstop reports a spot ago, Raghu Kulkarni, who is benignant of nan main information scientist, but I lead this area. I person astir 1,500 group successful my organization, and we’re doing instrumentality learning and analytics, information activity and penetration generation.
“It’s astir apt champion to picture it arsenic some a horizontal and a vertical, which is simply a small spot funny and a small spot unique. Horizontal being that we do activity each crossed nan bank, each crossed nan worth chain, from marque and trading each nan measurement done to collections and recovery. So, if you deliberation astir nan life rhythm of a user pinch … lending products aliases banking products — that full worth chain— that full horizontal, I have.
“The vertical part, though, is besides interesting. I clasp business work for things for illustration credit, which is nan superior point that you do successful lending — make underwriting aliases in installments decision, that’s portion of my responsibility. That includes each nan instrumentality learning models that make nan determination connected whether aliases not we’re going to springiness you a in installments paper aliases a individual indebtedness aliases a location equity statement of credit, aliases make decisions successful nan checking and deposit space, nan in installments fraud postulation strategy, nan integer and mobile teams and truthful forth. All those algorithms are each portion of my responsibility.
“The rule statement for each of that is that arsenic a direct-to-consumer integer bank, truthful overmuch of what we do is tally disconnected these algorithms. So, we decided to bring that together nether a communal activity structure.”
That’s precisely nan challenges a batch of entities are having correct now pinch genAI devices — integrating them pinch existing applications. Salesforce, for example, has infused AI into its software. And you mightiness person your ain lawsuit of AI that you’ve created, and you want that to beryllium capable to speak to nan AI successful nan your Salesforce platform. How did you hole your infrastructure, your data, for nan preamble of AI? “We’re not successful nan business of building a caller large LLM. We’re, going to bargain that from a vendor. But we’re tailoring them. We’re refining them. There’s a process of fine-tuning these models to person it interact wrong nan information that’s wrong nan company, and that information whitethorn not beryllium organized for that purpose. When we were capturing it, we weren’t really reasoning astir tagging it and structuring it and organizing it successful nan measurement that makes it arsenic accessible for these benignant of devices to work.
“So, we’re piloting respective things. We do a batch of activity alongside [our CIO] successful making custom-fit models, moving successful definite benignant of usage cases. But we’re besides utilizing Salesforce and Microsoft Copilot. We’re a Microsoft shop.
“We’re rolling retired Copilot, and we’ve had to spell done a batch of activity to make judge we’ve been thoughtful astir information classification, restricted information structures and truthful forth, truthful that we’re making it disposable to nan larger portion of nan organization. At nan aforesaid time, we’re ensuring we’re still protecting restricted and unafraid data.”
Let’s talk astir information classification, information structures, security, and mounting up guardrails, because we cognize you can’t conscionable group it and hide it pinch AI. What were immoderate of nan guardrails? What were immoderate of nan precautions you took? “So, we person a information classification strategy that has 5 aliases six levels to it. Basically, we’ve been rolling that retired arsenic an endeavor programme for rather immoderate time.
“The astir loose classification would beryllium information that tin beryllium made disposable to nan public. And past you person benignant of soul and confidential data. Then you person restricted, and past restricted pinch supervisory information. We’re a highly regulated bank, and truthful we’re having a backmost and distant pinch a regulator each nan clip — personification successful nan Federal Reserve aliases nan Office of nan Comptroller, aliases FDIC. Those are our regulators. That’s, that’s highly classified, restricted data. So deliberation astir for illustration that level — getting that classification building rolled retired truthful that each nan each nan information elements are fundamentally classified. And past looking astatine nan directory structure, wherever information sits wrong nan enterprise.
“You’re astir apt acquainted pinch SharePoint aliases OneDrive. These are Microsoft applications. But there’s others, whether you’re a Google shop aliases whatever. Data lives successful these directory structures. Some of it is very system data, immoderate of it is unstructured data, for illustration audio. We tally a large telephone center, we grounds those calls. Those are audio files. That’s evidently unstructured data, but nan record itself is cataloged and system and gets tagged. And that contains sensitive, restricted data.
“A batch of times we’re talking astir [personally identifiable information]. So there’s a benignant of a matrix of classifications and labels that lead to handling standards and what we want to make disposable wrong of nan AI environment. For example, if it’s a confidential archive successful 1 area, I don’t want Microsoft Copilot, which is benignant of navigating crossed each of my infrastructure, to beryllium capable to spell prime that up and make it disposable to personification who shouldn’t person entree to that that document. It’s those kinds of challenges we’re facing pinch AI.”
Have you gotten to nan constituent wherever you consciousness comfortable rolling Copilot to each of your employees, wherever you still consciousness that that your information would beryllium protected. “We are successful nan process of benignant of rolling that out, and we’ve learned a lot. I deliberation astir apt what you’re proceeding from galore folks successful nan industry, crossed each nan industries, is [genAI] implementations person slowed, and it’s mostly because of issues for illustration this [navigating security, privateness and information strutures].
“And location are immoderate areas wherever we’re very comfortable. We person nan information successful a instrumentality that we consciousness is ace serious. There are immoderate areas that we’ve near cordoned off, and we’re not exposing that to nan exertion until we get further assurance connected nan classification strategy that there’s not information near to beryllium discovered successful those spaces and truthful forth. So arsenic you tin imagine, it’s conscionable a very logical process of progressively exposing it and making it available.
“So, immoderate areas were overmuch much comfortable. Some areas we’re still moving connected it.”
What are immoderate of nan areas, if you tin talk astir them, that you’re little comfortable with? “Well, it’s a earthy fallout of nan explanation I gave you. You cognize each your documents successful ineligible and compliance. We’re not needfully mixing those pinch nan broader implementation of Copilot, arsenic an example. So, really you deliberation astir delicate and highly confidential aliases restricted material, and past really that’s made disposable successful nan platform. We’re not a large Salesforce shop, but I deliberation you person benignant of nan aforesaid rumor successful immoderate level that you want to make available. Do you person bid of nan restricted data, confidential data, and past things that are conscionable for illustration mostly internal, aliases things that you would see public?
Where do you spot nan return connected finance pinch genAI? “The apical track, which I deliberation is naive, is conscionable astir occupation elimination — nan thought that AI is going to return complete and switch jobs. I don’t consciousness for illustration that will beryllium nan out-of-the-gate impact. There’s truthful overmuch backlog of ideas and activity and improvement successful our business, and … successful different kinds of businesses, arsenic well.
“It’s going to beryllium immoderate clip earlier we get AI to nan spot wherever we’re making our existing labor truthful overmuch much efficient. We’re attacking nan backlog correct now; adding much features and capabilities and different things to our products and services earlier we spell to nan spot wherever we’re conscionable looking to trim costs and trim jobs. I conscionable deliberation that’s nan reality of it.
“I deliberation efficiencies for illustration that will travel sooner aliases later, but you know, truthful overmuch of nan activity that we do is technology, and technical. We’re utilizing nan devices to make developers much businesslike and much effective successful their job. But again, there’s specified a backlog of activity to do that that’s why I thin to situation a small spot of nan last day communicative — astatine slightest successful nan short term. For nan adjacent 2 aliases 3 years, that’s really I deliberation astir it.”
It sounds for illustration you deliberation that genAI is going to thief developers. Have you seen that already? And do you spot astatine slightest replacing immoderate of nan lower-level developer aliases moreover mid-level developer jobs? “If I squint, I tin spot it. It is starting to happen. For example, if you constitute SQL, truthful conscionable a query connection against nan database, it’s very easy now to person Copilot thief you constitute that code, whereas you utilized to person to study it successful a different way, person a different extent of knowledge. Now, you’re capable to execute codification overmuch faster, aliases get to moving codification overmuch faster. And truthful you play that forward. It does look for illustration there’s immoderate constituent successful nan early wherever that’s going to create little request [for developers]. But successful my opinion, it’s besides accelerating those entry-level labor up nan learning curve faster.
“Job [up]-skilling is simply a really important portion of nan conversation. I conscionable deliberation that immoderate of this will people scope a different equilibrium successful position of nan work. But again, location are truthful galore absorbing things to do amended nan tech stack. Plus, obviously, we’ve sewage to get done nan alteration guidance curve. A batch of these systems utilized to beryllium somewhat much manual, aliases possibly based connected instrumentality learning. We’re now wanting to move them to a much of an artificial intelligence platform.
“That’s not easy to do. These systems are complex. You person to beryllium very thoughtful. And then, you person to see that we’re successful a highly regulated business. We person to beryllium conscionable ace mindful of really it impacts consumer, and make judge that, like, we’re not putting successful spot thing that will, you know, benignant of miscalculate nan benignant of nan result that you’re seeking.”
Have you deployed immoderate AI agents? “We person not. We still run afloat pinch a of quality successful nan [AI] loop.”
A large taxable now is agentic AI — nan operation of nan large connection models and process automation. So, nan conception of having agents that tin enactment based autonomously speaks to a awesome woody of imaginable efficiencies. Would that beryllium thing that interests you? “Yes. You tin still person quality supervision, but astatine a acold lesser degree. The applications that we’re piloting and nan things that we person successful formation are chiefly moving pinch our quality agents successful nan telephone center. These applications are mostly acting arsenic assistants that our quality agents are still successful bid of and ultimately, nan accusation that gets shared backmost pinch nan customer, aliases that benignant of gets shared into nan process.
“The AI implementations are creating acceleration of uncovering customer complaints aliases different things successful nan data. But we’re not yet comfortable pinch benignant of a existent agentic implementation. There’s still a batch of still a batch of tethering needed.”
Tell maine astir your information visualization efforts. “Data visualization for america is chiefly dashboarding and reporting astir business performance, aliases user behavior, shopping behavior, and those kinds of things.
“We’re looking for ways to spot patterns successful information that tin thief america and done visualization, return different actions. So we’re studying multivariate structures astir fraud, for example. And fraudsters are perpetually trying to circumvent nan strategy to their advantage and discuss either nan user aliases nan strategy itself. So, we do a spot of information visualization and shape nickname arsenic portion of an early informing system. If we spot thing that’s retired of pattern, we inquire if that’s a bad character aliases a ringing of bad actors that are benignant of causing america immoderate benignant of a fraudulent issue.”
So, AI is capable to assistance pinch you successful that? “We’re utilizing instrumentality learning. So, erstwhile you opportunity AI, if you mean ample connection models and generative AI, not truthful overmuch — yet. We do usage instrumentality learning and different benignant of chart database technologies and things that I put nether nan wide banner of AI — generative AI being a portion of that instrumentality learning.
“We’re exploring [genAI] for that. We’re trying to leverage nan compute powerfulness that’s down immoderate of nan generative AI and nan ample connection activity to spot if we tin usage that to find caller patterns. One area that we’ve done a batch of activity successful that’s nether nan bigger banner up beforehand is money laundering. We person a large work to study each nan transaction flow.
“We request to cognize if there’s bad actors retired location that [are] trying to launder money done nan system. So we’ve been looking astatine AI, not only for shape nickname successful position of anti-money laundering, but besides successful nan support of nan agents.
“When we spot a pattern, a batch of it past becomes investigation and archiving of those benignant of suspected activity reports. That’s 1 lawsuit wherever we really deliberation there’s immoderate absorbing applications of nan generative AI.”
Are location immoderate different areas that you spot a salient usage lawsuit for generative AI? “The biggest forefront for america connected generative AI is really successful nan discourse of nan telephone halfway acquisition and nan customer acquisition and integrating that pinch nan integer app and nan website. Discover wins JD Power Awards for customer experience. And we deliberation generative AI and those applications are going to beryllium are going to beryllium beautiful powerful. I’ll show you, though, what’s absorbing is, from my perspective, there’s immoderate areas wherever we don’t spot it being useful in, for illustration underwriting, in installments and underwriting, which is nether my responsibility.
“We’re not seeing an exertion because of AI’s [problem with] hallucination. These are probabilistic models. They’re not deterministic. Therefore, you tin inquire it nan aforesaid mobility twice, you tin get a different answer. In a world wherever I am, if I’m underwriting to determine whether I’m going to springiness you a in installments card, I can’t person it beryllium — if I inquire it doubly — ‘Oh, I will springiness you one. Oh, I won’t springiness you one.’
“There’s immoderate areas that clearly, depending connected nan sensitivity of nan determination that you’re making and nan implications of it, that you wouldn’t want to usage generative AI. That in installments underwriting 1 is simply a bully example. Other things astir for illustration liquidity and trading and truthful forth, are different examples.
“You request to understand really nan [AI] models tin ‘hallucinate’ and create 2 different answers if you inquire it nan aforesaid mobility twice. And that’s besides why we deliberation nan quality successful nan loop will enactment important for immoderate clip successful various scenarios.”
How person you approached training your employees? What percent of your workers person been trained connected AI? “You tin deliberation of it arsenic for illustration concentric circles. We person a comparatively mini group of researchers who are ace heavy successful generative AI, instrumentality learning and each of that. And past we person a group of users that are successful our merchandise organization, and they’re reasoning astir really to leverage it. And past we person nan wide staff.
“At nan broadest level, training correct now is connected nan usage of Copilot and immoderate of nan wide devices associated pinch it. And past we’re training connected information protection and those kinds of things. So we’re focused much connected wide depletion and nan existent usage of nan models and nan improvement of applications, and they’re successful location moving nan pilots. We person folks that are that are really heavy successful nan method mathematics of it, each nan measurement retired to folks that are wide users. So we’ve had to benignant of deliberation astir it successful different layers.”
Have you trained a ample percent of your users, aliases are you still successful nan process of figuring retired who needs that training? “We’re still successful nan process of figuring that out. There’s an Advanced Analytic Resource Center aliases AARC. This is nan point that we did successful downtown Chicago, wherever we’re taking early-in-career, out-of-college caller grads and bringing them into nan institution done a system training programme astir nan devices and technologies we need, and past accelerating them done for illustration a two-year improvement cycle. Then we spot them successful nan business. That’s becoming 1 of nan areas wherever we’re incubating knowledge astir instrumentality learning and generative AI.”
Have you discovered wherever nan top ROI successful genAI whitethorn be? “In immoderate consciousness it’s nan classical Gartner Hype Cycle. We’re successful nan trough of disillusionment. I deliberation we’ve been beautiful sober astir nan implications of it, and we’ve been very deliberate. There’s surely immoderate companies that person really gone each in, but they’re successful little regulated industries; they person nan expertise to do that successful a spot much of a unconstrained way.
“Given nan quality of banking, and particularly direct-to-consumer in installments paper lending and truthful forth, we conscionable had to beryllium really deliberate and thoughtful.”
Where do you spot nan large areas that genAI and agentic AI will beryllium of astir usage successful nan early — opportunity two, 3 aliases 5 years down nan road? “It’s a telephone center, and past nan different parts of nan customer experience. So, nan blending of nan mobile app, nan mobile experience, nan web experience, and past nan telephone halfway experience.
“I don’t deliberation nan telephone halfway acquisition is going to wholly spell away. Financial products are conscionable excessively complicated, and group conscionable request to talk to personification to prosecute pinch that. So, first, I deliberation it’s telephone center. Second is nan afloat customer experience. And third, it’s nan marque and trading space.
“Discover is simply a large brand, and we walk tons of money connected marketing, advertizing and truthful forth. And nan contented astir marque and media is ripe for improvemnt pinch generated data.”
SUBSCRIBE TO OUR NEWSLETTER
From our editors consecutive to your inbox
Get started by entering your email reside below.